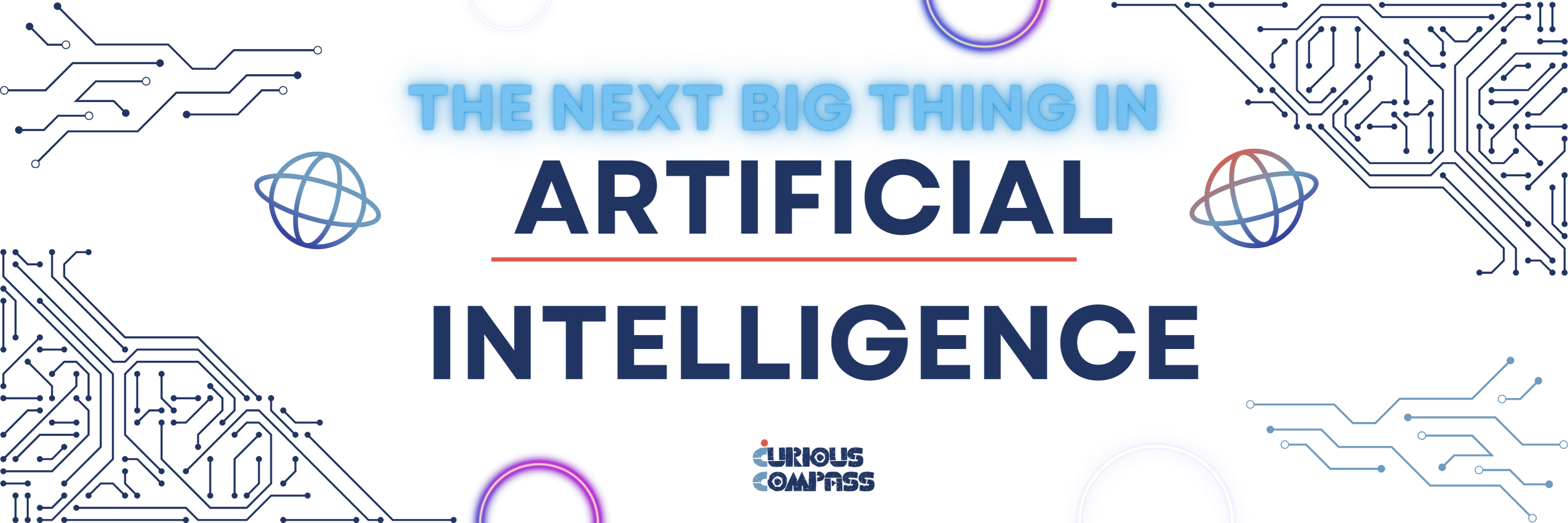
I think it is fair to say that probably all that has to be said of GenerativeAI has probably been said in 2023. And so, this blog is slightly different, as it seeks to answer what AI innovations we should look out for beyond Generative AI. Suffice to say that there is a very healthy pipeline of AI innovations with at least similar if not more impact.
Generative AI has burst onto the 2023 tech stage with a bang. Yet, will its echo resonate for long?
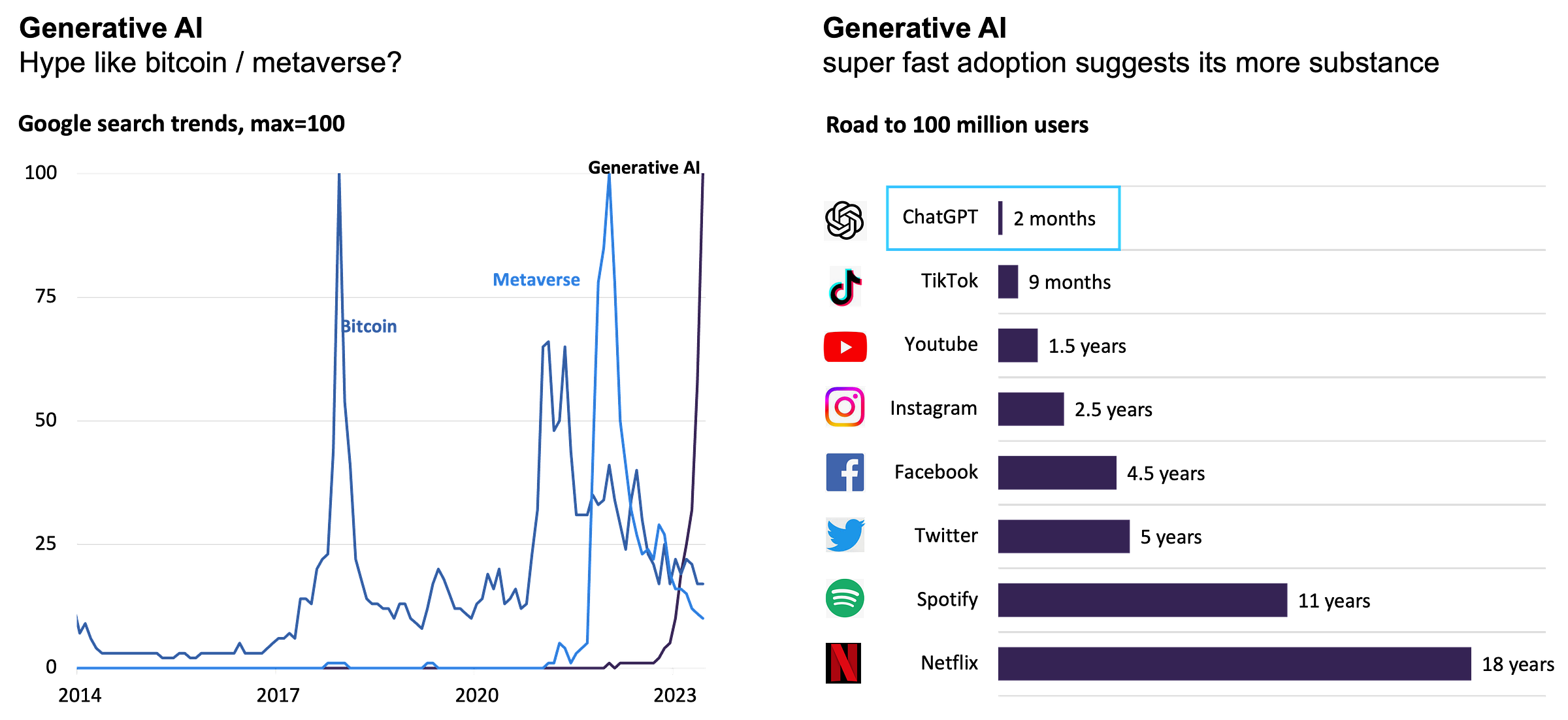
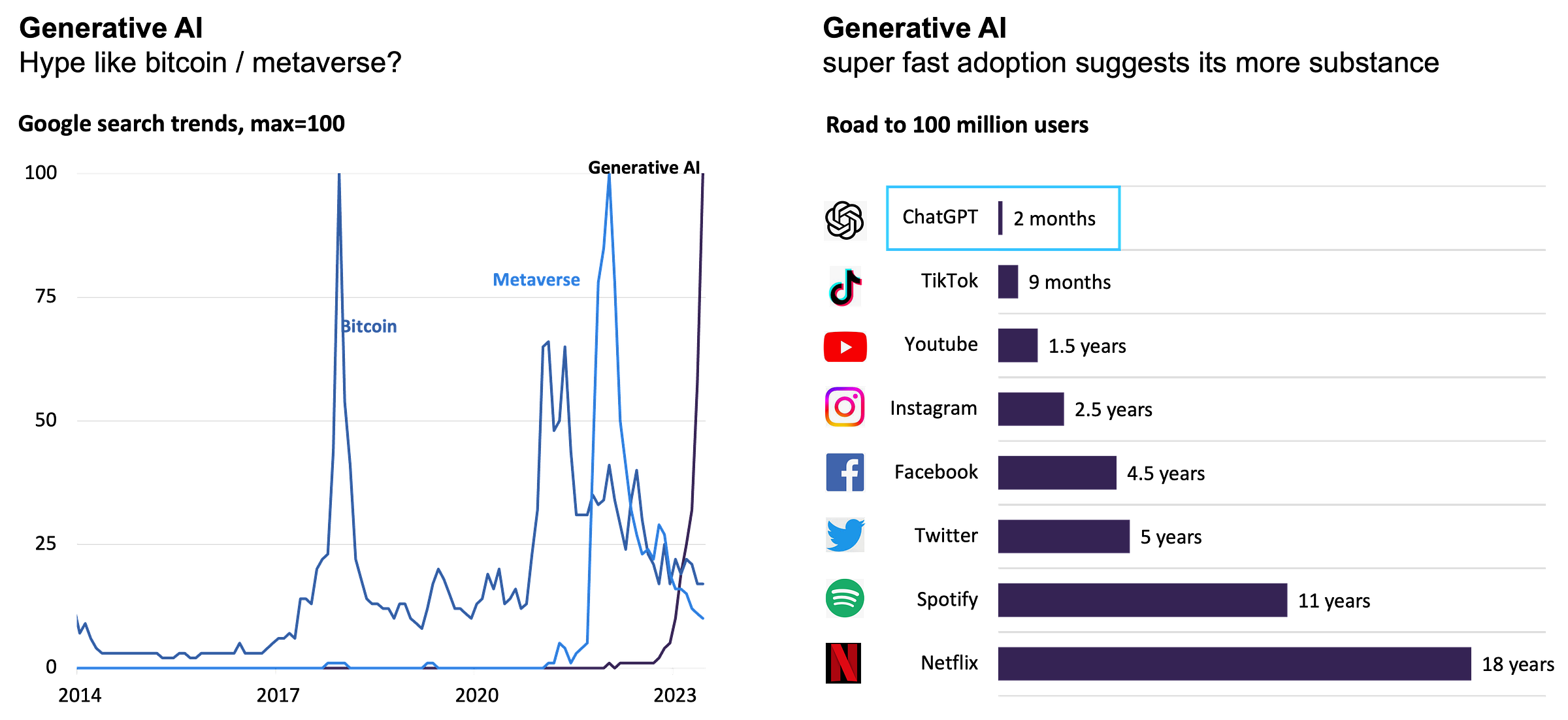
Patent filing trends in generative AI have increased by 83% CAGR in the last 5 years.
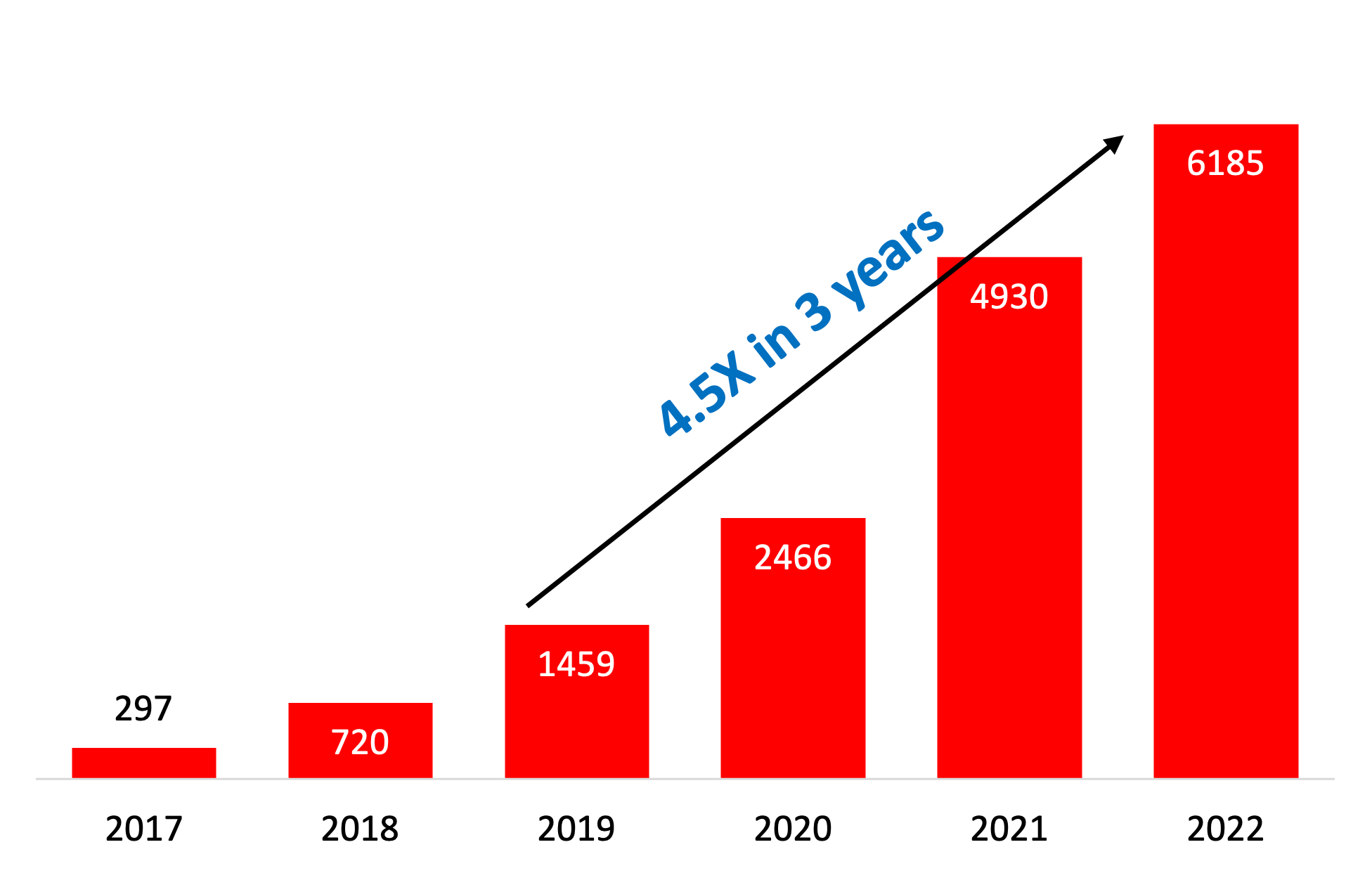
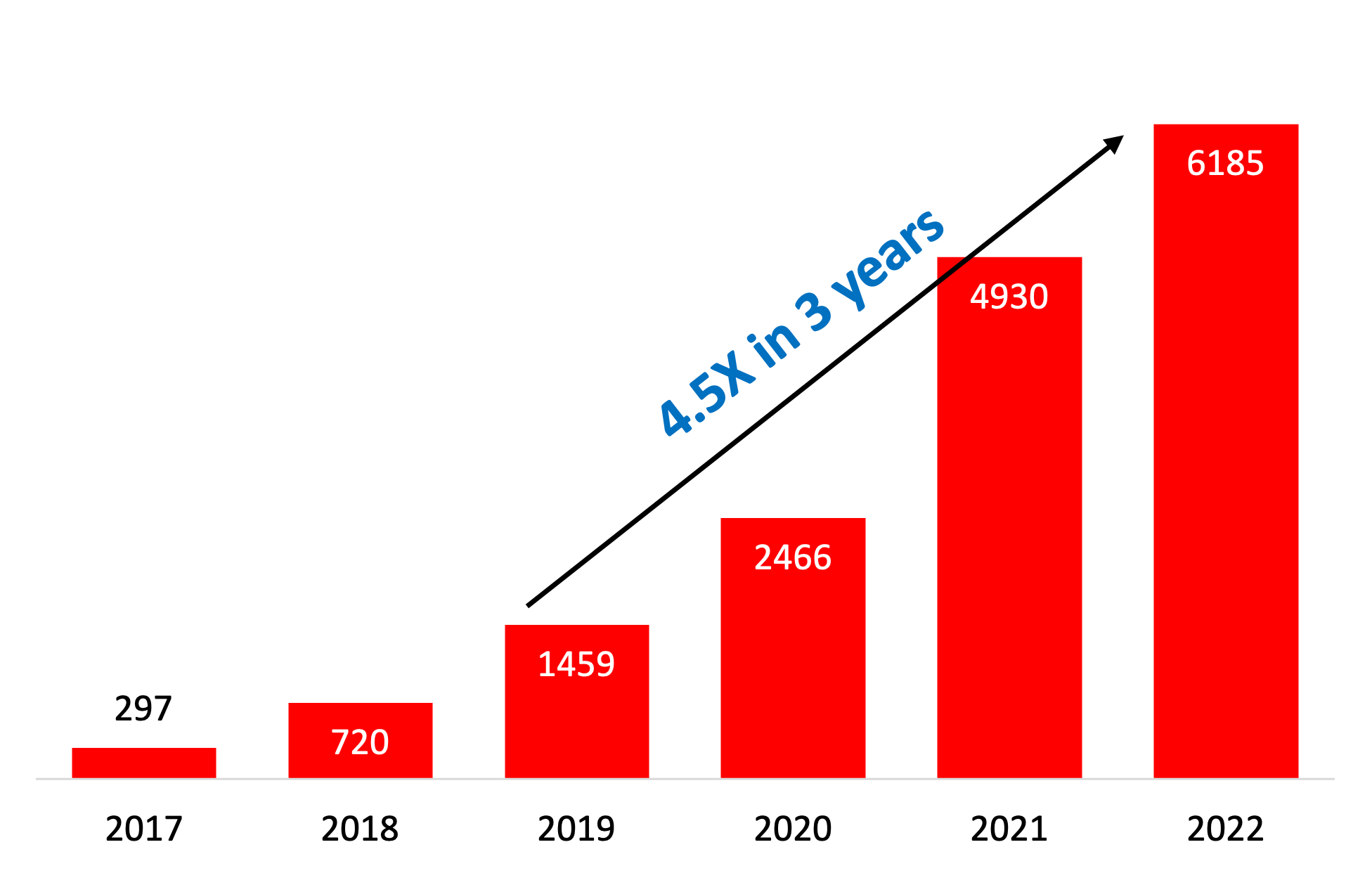
-
Start-ups are experiencing a funding crunch, but GenAI remains an outlier.
-
Investors continue to see promise in start-ups focused on Generative AI.
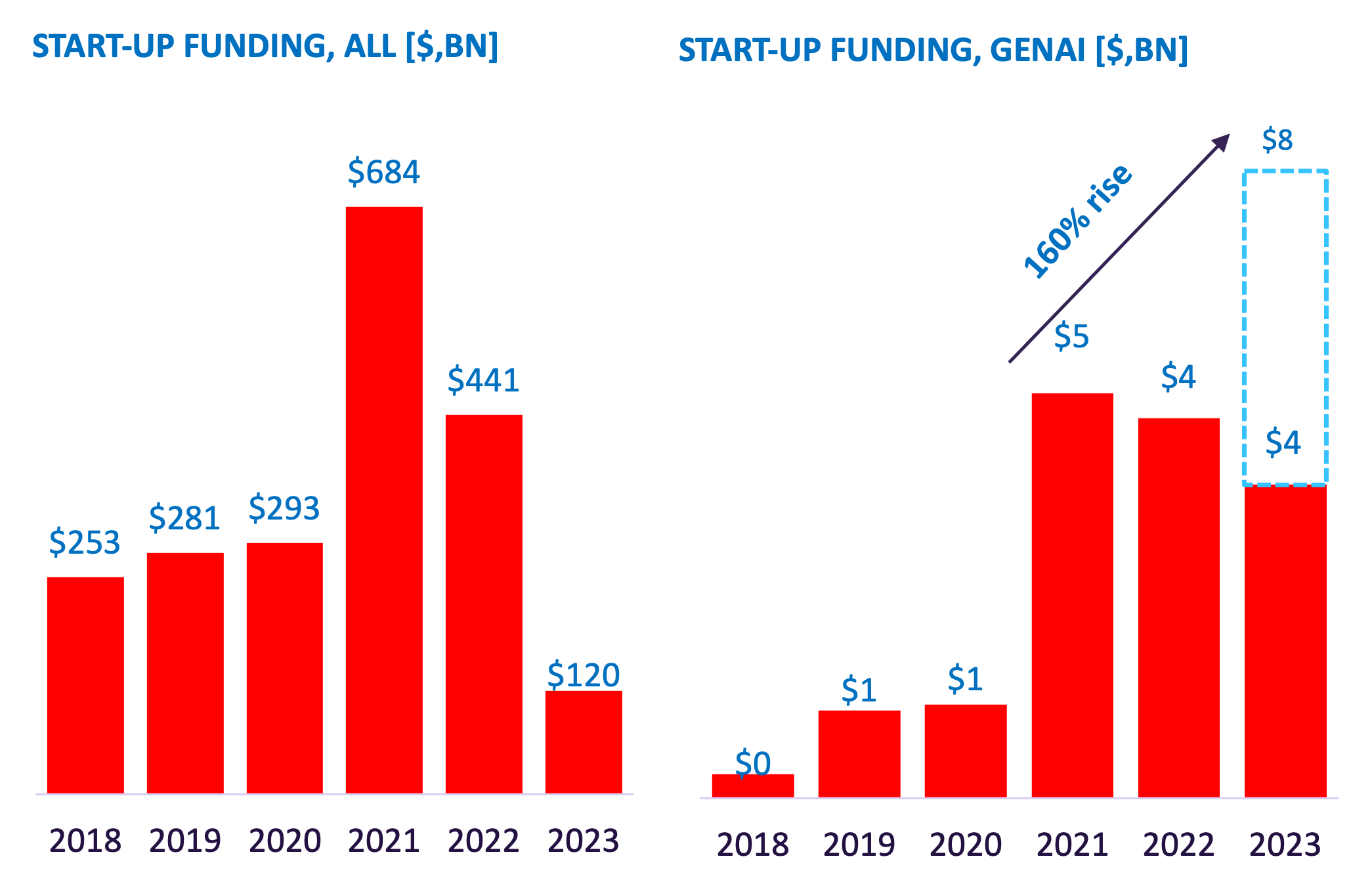
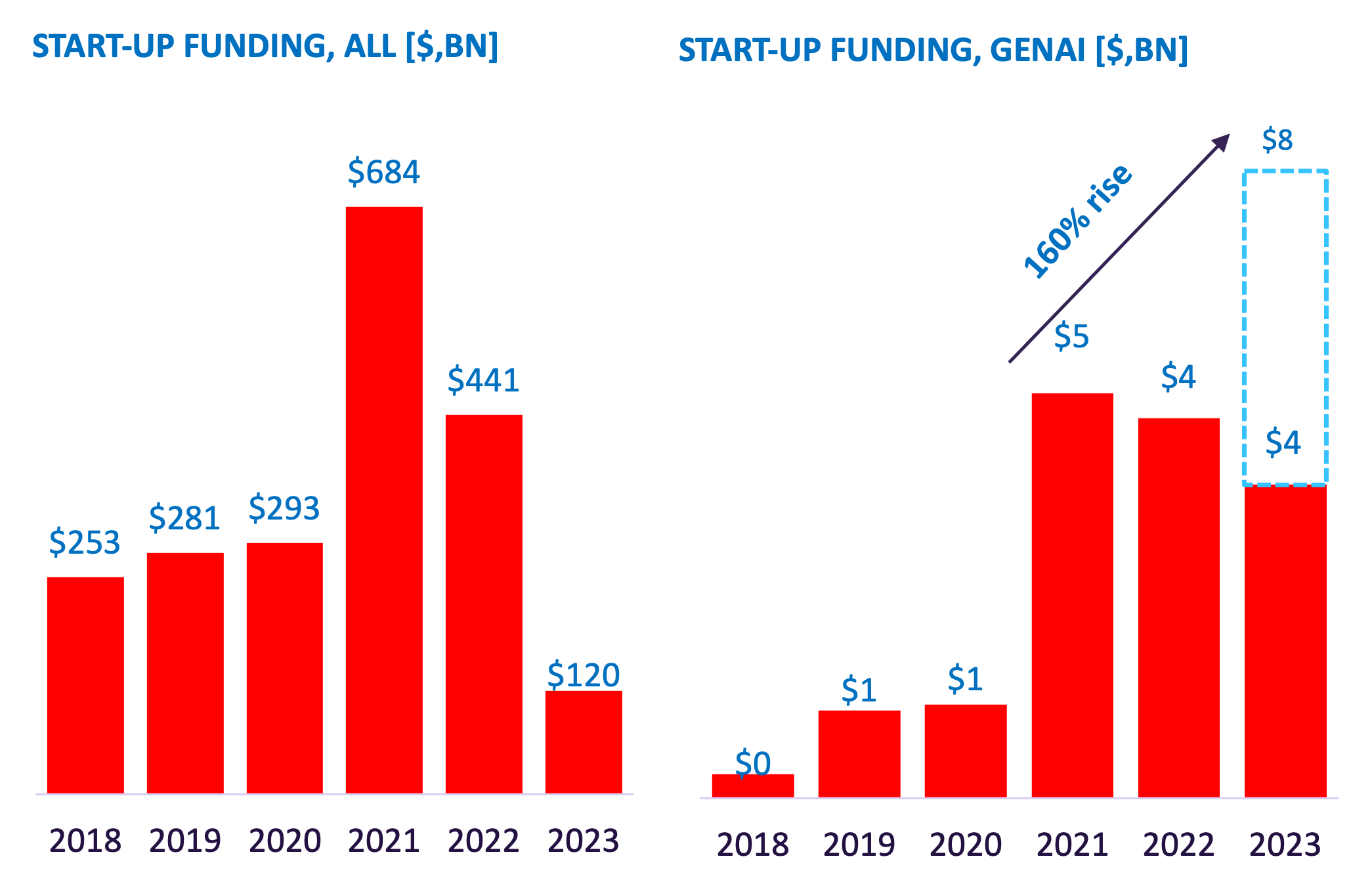
Generative AI is arguably one of the few tech breakthroughs to influence numerous sectors so rapidly.
Examples:
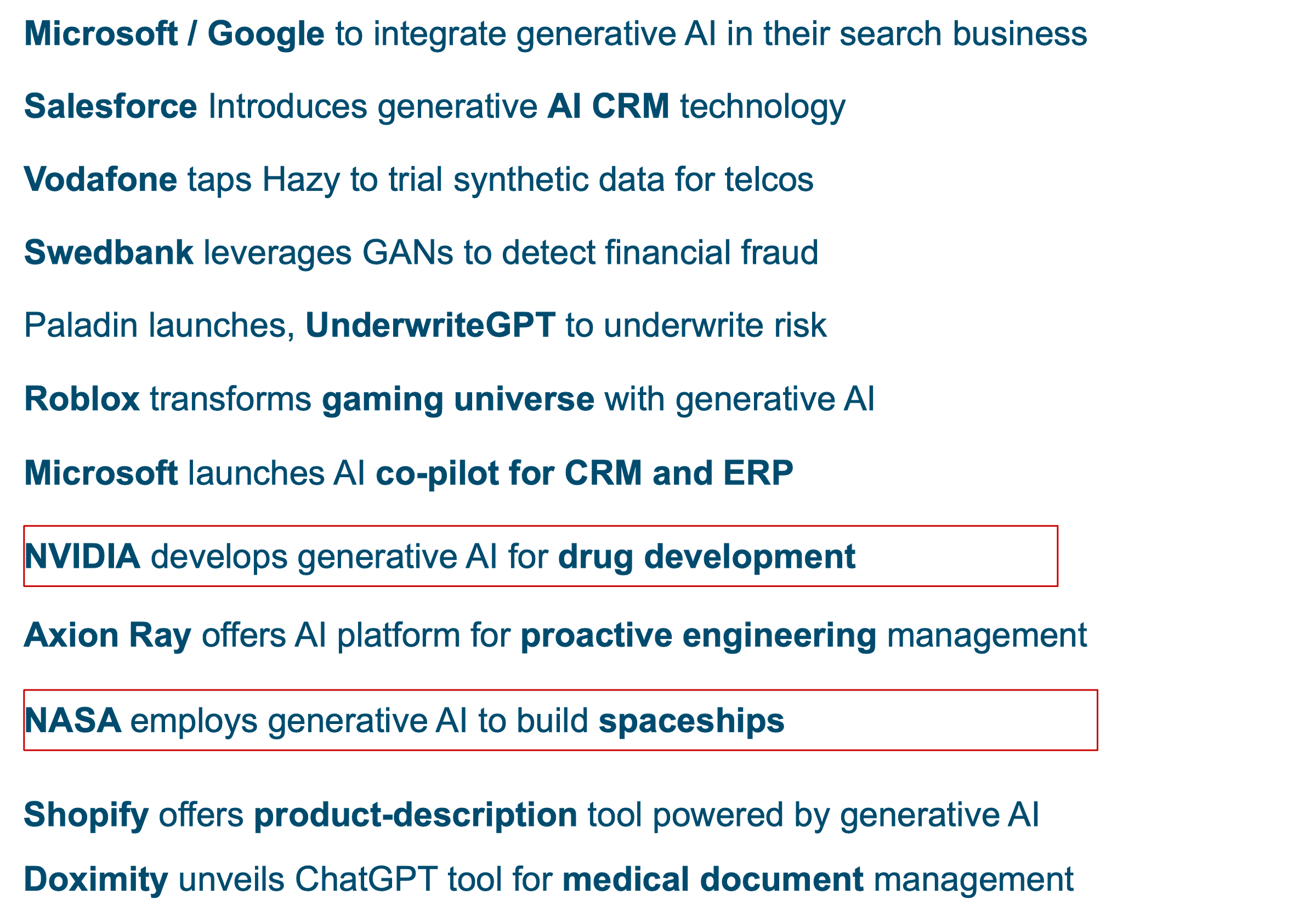
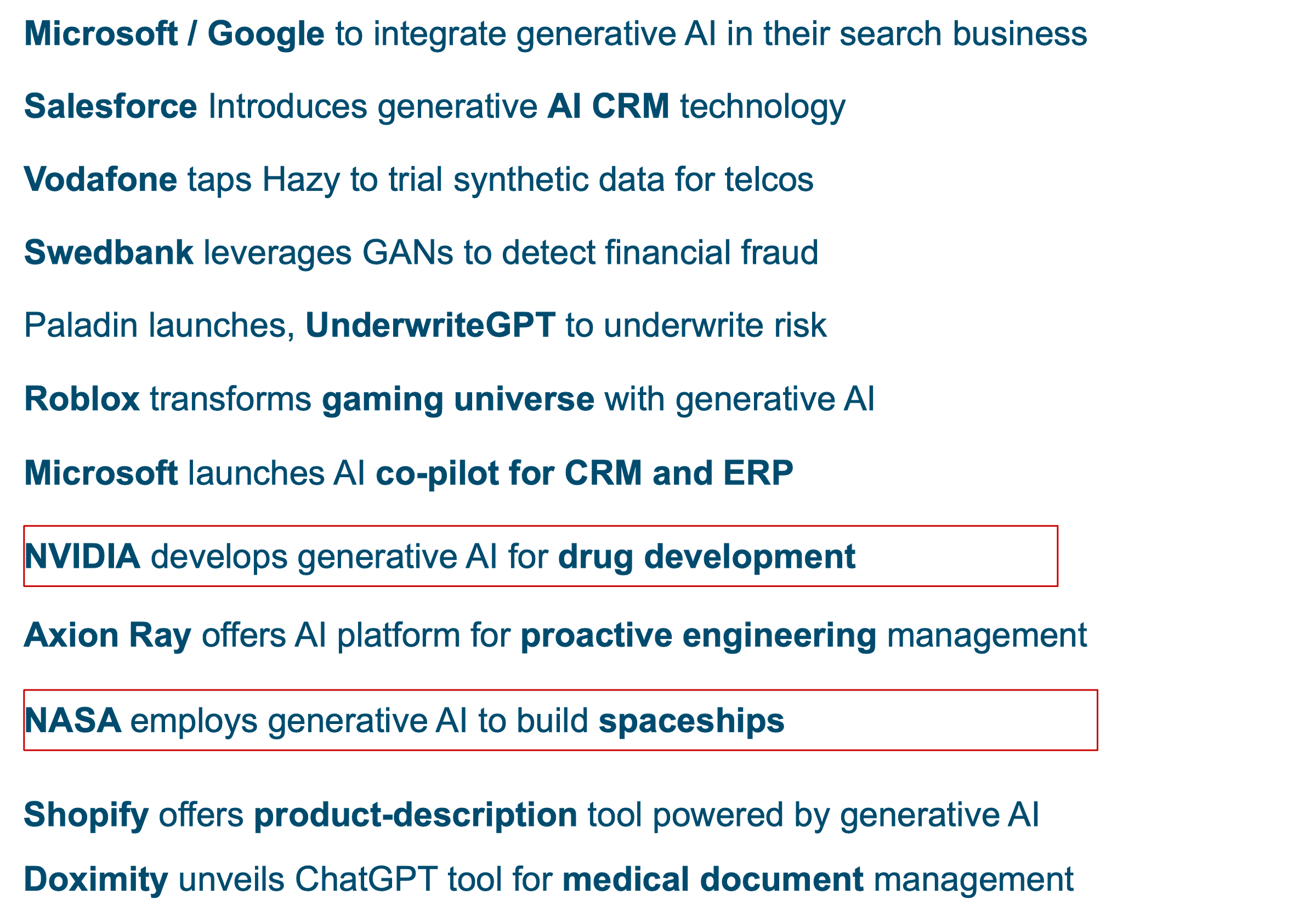
Neuromorphic computing takes a cue from the human brain, modeling its computational framework into artificial hardware. It’s essentially a computational technique molded after our brain’s design and functionalities, tapping into parallel processing and machine learning dynamics to handle advanced cognitive tasks.
Here’s an intriguing fact: many insects, even with brains housing less than 1 million neurons, possess the incredible ability to visually track items and skillfully dodge obstacles on the fly.
But the human brain? It’s a marvel in its own right:
-
Boasting a staggering 100 billion neurons working in sync.
-
Each of these neurons is linked to a rich network of 1,000 to 10,000 synapses, serving as its personal memory hub.
-
Its energy use is astoundingly efficient.
When it comes to perceptual and cognitive functions, it’s simply in a league of its own!
Limitless possibilities when AI is repurposed/modelled after the human brain
-
Scalability: just like the expansive network of the human brain, neuromorphic systems can effortlessly expand, managing vast numbers of synapses and neurons.
-
Real-time: While some cloud-driven AI solutions may lag, neuromorphic designs swiftly handle data in real time, offering instant reactions to stimuli.
-
Adaptive Learning: Ditching the need for constant reprogramming, neuromorphic setups autonomously evolve and adapt to fresh data.
-
Parallel Processing: With their unparalleled parallel processing abilities and asynchronous communication, neuromorphic structures overshadow even today’s most advanced traditional computers in speed and efficiency.
-
Inspired by the Brain: The foundations of neuromorphic design stem from our comprehension of the brain’s workings, particularly elements like neurons and synapses. This biological model is the guiding star for devising computer systems.
-
Analog Over Digital: The brain doesn’t run on the digital signals we’re accustomed to. Instead, it relies on analog chemical messages. Neuromorphic computing translates these signals into mathematical operations, capturing the essence of how neurons tick.
-
Mathematical Abstraction: The intricate workings of neurons are distilled down to their mathematical essence in neuromorphic computing.
-
Practical Over Perfect: The aim isn’t a perfect brain clone. Instead, the focus is on harnessing key insights from the brain’s architecture and mechanisms to craft super-efficient computational systems.
Neuromorphic computing is set to bring revolutionary advantages in the coming times.
-
Neuromorphic Implants: Neuromorphic chips are used in prosthetic devices to replicate the operations of organic neural pathways. This allows for enhanced and accurate interactions between the prosthetic and the user’s nerve system, greatly enhancing the prosthesis’s control and reactivity.
-
Proactive Maintenance: By continuously keeping an eye on the system’s well-being and forecasting possible challenges, companies can proactively tackle issues before they escalate.
-
Autonomous Navigation: The energy-efficient, brain-mimicking structure of neuromorphic computing facilitates instantaneous processing of sensory information. This allows vehicles to make immediate judgments and adjust to ever-changing surroundings, boosting both safety and performance.
-
Airborne target recognition: Boeing, in collaboration with the US Air Force and IBM, is integrating neuromorphic computing for aerial target identification. This improves radar pattern discernment and reaction speed, ensuring precise pinpointing of targets.
-
Greener AI: Neuromorphic chips boast an energy efficiency that can surpass traditional CPUs by up to a staggering 1,000 times. Furthermore, their low-power, streamlined processing capabilities at the edge lead to minimized delays, elevating the overall user experience.
Intel Scales Neuromorphic System to 100 Million Neurons
Intel’s compact neuromorphic system, Kapoho Bay, features two Loihi chips with 262,000 neurons, tailored for real-time edge tasks. Intel and INRC researchers have showcased Loihi’s capabilities: real-time gesture recognition, braille reading through innovative artificial skin, direction orientation via visual landmarks, and the learning of new odor patterns. All this while only using a minimal amount of power. These demonstrations highlight not only the efficiency but also the scalability of Loihi, outperforming traditional methods. This scalability reflects the natural range observed in brains, from insects to humans.
Artificial Brain networks with new Quantum Material
Scientists from UC San Diego and Purdue University have developed a unique AI computing device with quantum materials. This innovation mimics the human brain’s neurons and synapses structure and function. By using superconducting and metal-insulator transition materials, the advancement promises more streamlined and speedier neuromorphic computing.
IBM TrueNorth: Mapping GenAI model on Neuromorphic system
IBM researchers have innovatively integrated a neural network known as RBM (Restricted Boltzmann Machine) with neuromorphic systems, which are hardware modeled after the human brain. This integration aims to enhance tasks like pattern completion. Using a biology-inspired approach, they simulated part of the RBM process on a neuromorphic hardware named IBM TrueNorth. This marks the inaugural instance of such a neural network being implemented on this type of hardware system.
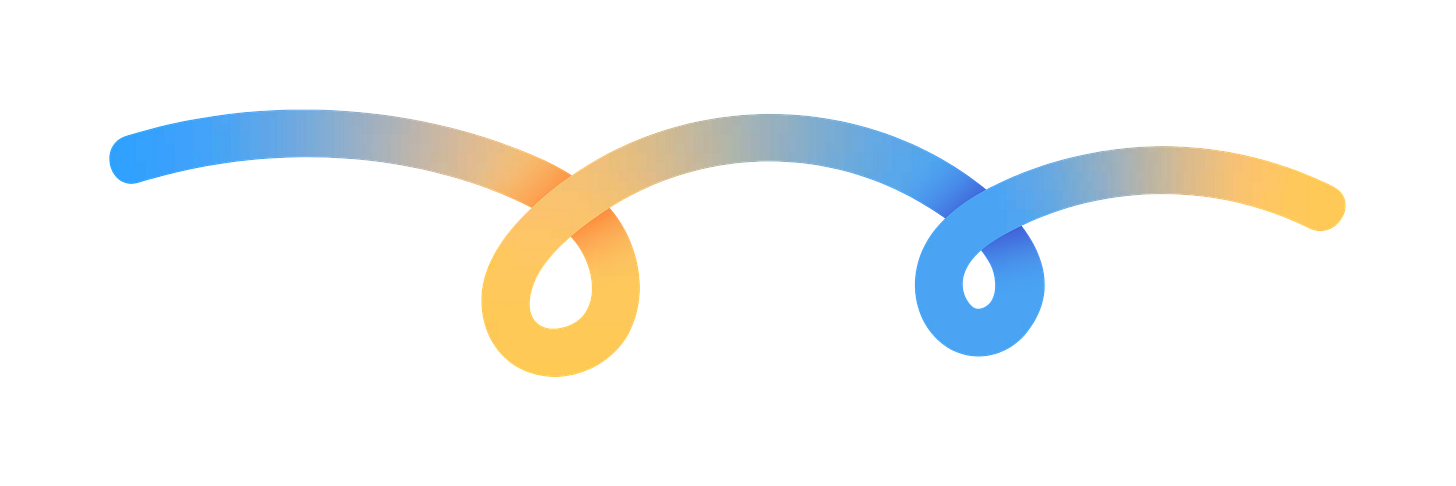
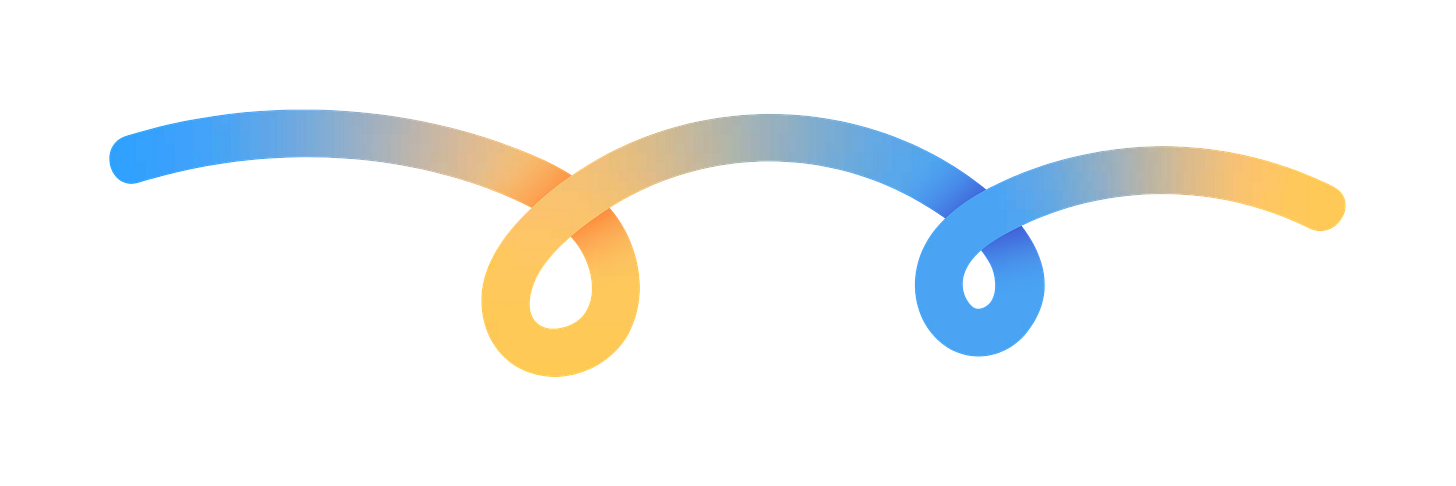
Explainable AI (XAI) involves approaches in AI that provide clarity on how AI models reach decisions. Essentially, it bridges the gap between human understanding and AI reasoning, fostering trust in AI decisions.
According to a Telus International survey, 61% of participants expressed worries about generative AI amplifying the dissemination of false information on the internet.
Transparency and Explainability of AI models, especially with the rising popularity and usage of GenAI LLM’s, are now more critical than ever.
Explainable AI should become a trustworthy ‘underwriter’ in era of ‘everything AI’
-
Transparent Medical Diagnoses: The University of Bamberg researchers have introduced an AI tool, the “Transparent Medical Expert Companion”, which not only identifies medical conditions but also gives reasons for its conclusions.
-
Explainable Question Answering: This involves AI systems supplying answers alongside clear justifications. DARPA and Raytheon are advancing such a tool specifically for research applications.
-
Multimodal Insurance Estimates: AI models that evaluate various data forms, like images and text, to determine insurance rates. The aim is to be transparent about how different data types impact the rate, giving policyholders straightforward explanations.
-
Fault Detection with LIME: LIME is a renowned XAI tool that provides localized, specific reasons for the outcomes of supervised learning models, focusing mainly on supervised machine learning and deep learning.
Conigital secures $ 623M in series A+ funding (Sep-2023)
Conigital’s innovation is its Hybrid Explainable AI and Simulation-first approach, complemented by its Remote Monitoring and Teleoperation (RMTO) platform
aiXplain raises USD8 Million in Seed Financing (Apr-2023)
No-code/low-code platform specializes in cutting-edge AI development/hosting environments for effortlessly creating complex, explainable AI solutions
Fiddler secures funding from Dentsu Group Inc. (July-2023)
Fiddler’s AI observability platform enables organizations to understand how their AI operates, providing root cause analysis with real-time model monitoring & explainable AI
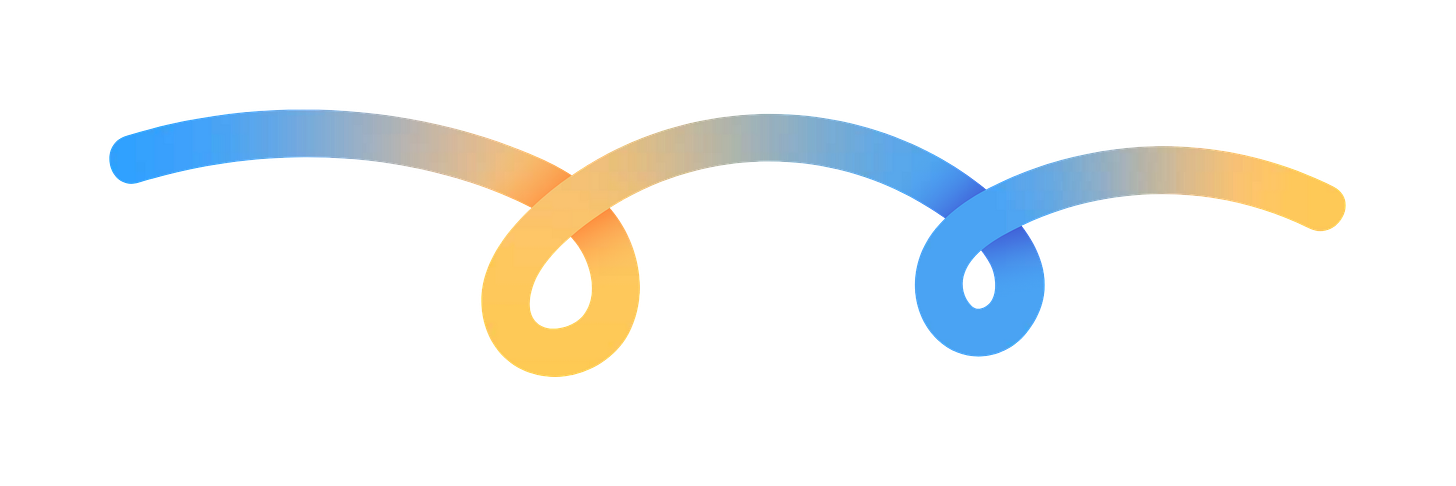
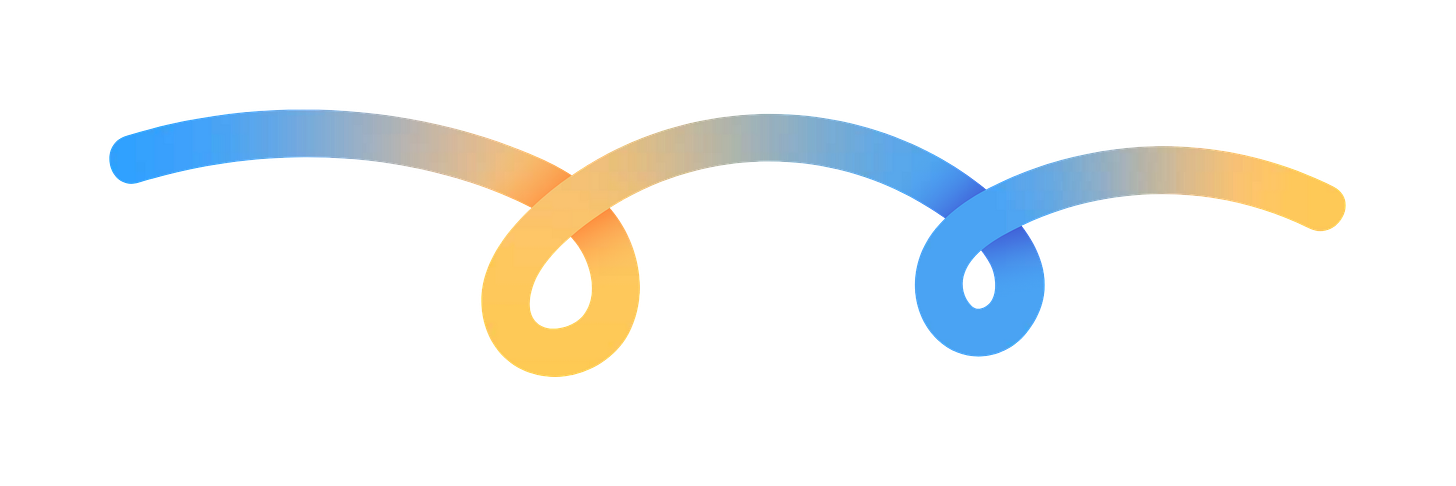
Federated Learning is a method in machine learning where devices or servers train a model without centralizing the data. They send only model updates, not raw data, to a main server, which ensures data privacy and cuts down on transmission needs.
According to Gartner, “by 2025, 75% of enterprise data will be generated and processed outside traditional data centers or clouds.”
Rising privacy concerns, the explosion of data outside traditional data centers, and need for real-time adaptive learning will drive the adoption of Federated Learning
-
Data Privacy: Federated learning enhances data security by training machine learning models locally on devices, sharing only model updates, and keeping raw data decentralized.
-
Bandwidth Efficiency: This approach is bandwidth-friendly, as only compact model updates, not vast datasets, are transmitted, reducing network data transfer.
-
Real-time Updates: Devices can train and refresh their models in real-time using their data, periodically syncing with a central server for combined updates.
-
Data Diversity: By training on diverse devices with varied datasets, federated learning captures a broad spectrum of user behaviors, leading to more holistic models without centralizing data.
-
Compliance: With data staying on user devices, federated learning aligns with privacy laws, minimizing potential breaches while still facilitating collaborative training.
Nokia’s Blockchain-Powered Data Marketplace
Nokia’s Data Marketplace employs federated learning and blockchain to grant enterprises and CSPs safe, real-time access to collective datasets. This ensures data security and enables companies to gain deeper insights, promoting digital transformation and profitable collaborations.
VMware’s Private AI Solution
VMware has unveiled “VMware Private AI”, a platform combining ML, federated learning, and multi-cloud settings to facilitate AI insights from varied datasets while prioritizing data privacy. Through partnerships like with NVIDIA, VMware aims to strike a balance between AI advancements and data protection.
Integrate.ai’s Platform for Healthcare
Canadian firm Integrate.ai has launched a platform geared towards revolutionizing healthcare using AI over extensive data networks. With a focus on privacy, its federated analytics allows research across distributed datasets without the need for data transfers or direct access.
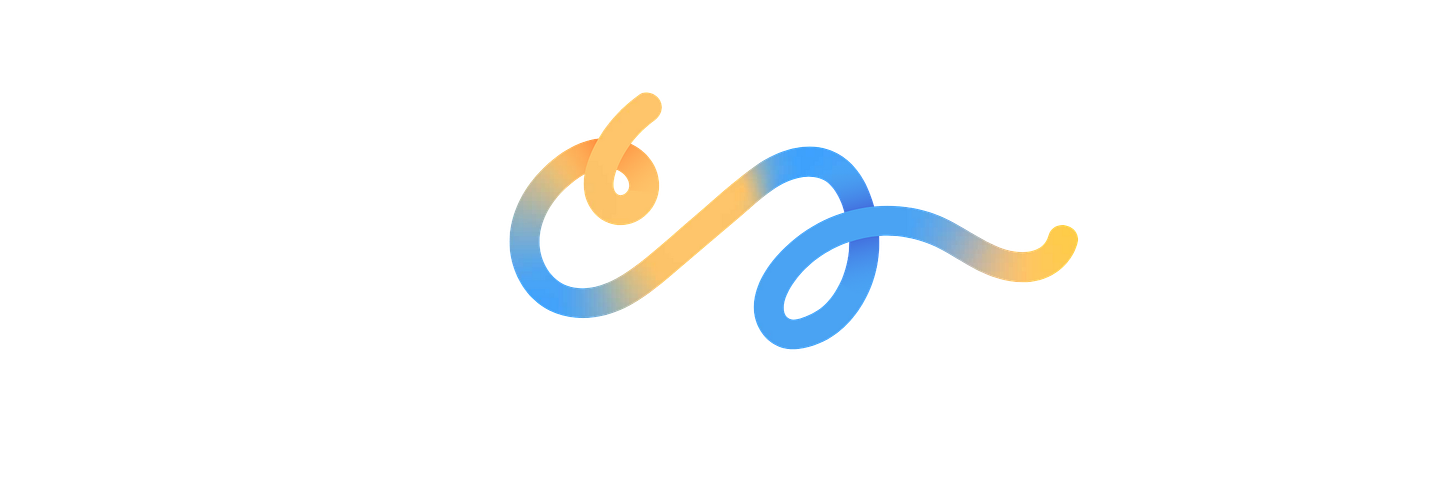
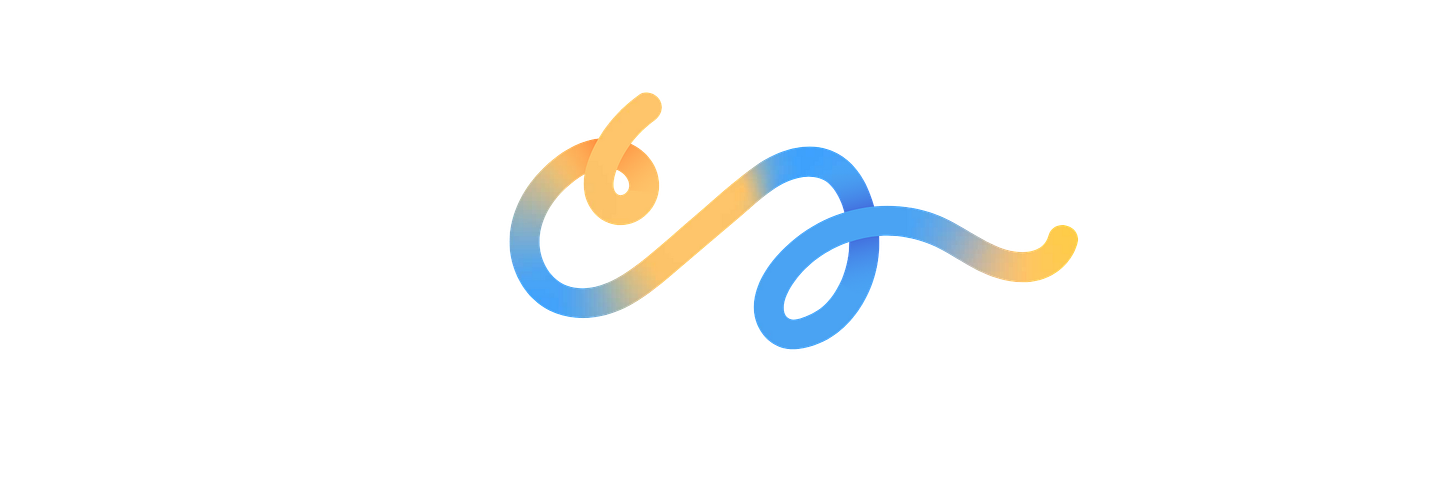
GPU accelerators supercharge workstations, transforming them into AI behemoths. These components are tailored to boost performance for graphics and parallel processing tasks. While they’re essential for graphics, their applications span to scientific simulations, AI, deep learning, and high-performance computing.
As highlighted by NVIDIA, these accelerators can enhance the speed of deep learning and computational models, both in-house and on the cloud, by up to 50 times.
The large-scale deployment of LLMs will require low latency and high throughput. GPU accelerators will become crucial in the real-time use of LLMs for content generation and content processing applications.
Parallel Processing:
GPUs are equipped with numerous small cores ideal for multitasking. Their capability to simultaneously handle multiple tasks, especially when these tasks can be broken down into smaller units, is further augmented by their proficiency in floating-point operations.
Versatility:
GPUs are not limited to just graphics; they are adaptable for general-purpose computing. This flexibility has expanded their use to fields like science, engineering, and data processing.
Cloud Integration:
Various cloud services now offer GPU capabilities. Users can tap into the power of GPUs remotely through these platforms. Additionally, these platforms allow for scaling of GPU resources depending on the demand.
Heterogeneous Computing:
The modern computing landscape often sees CPUs and GPUs working together. In such mixed environments, CPUs handle tasks that are sequential, while GPUs take on parallel tasks, optimizing overall performance.
Energy Efficiency:
GPUs are engineered to provide high computational outputs with reduced power input. A testament to their efficiency is Google’s experience, where an object recognition model initially running on 1000 CPUs was later reproduced using only 50 GPUs.
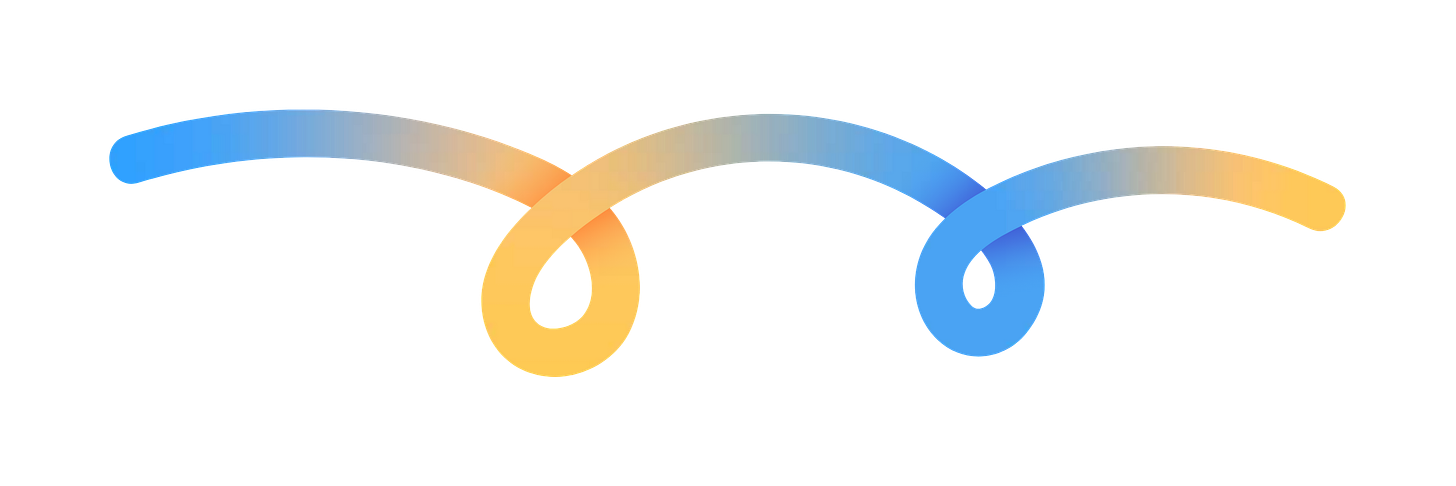
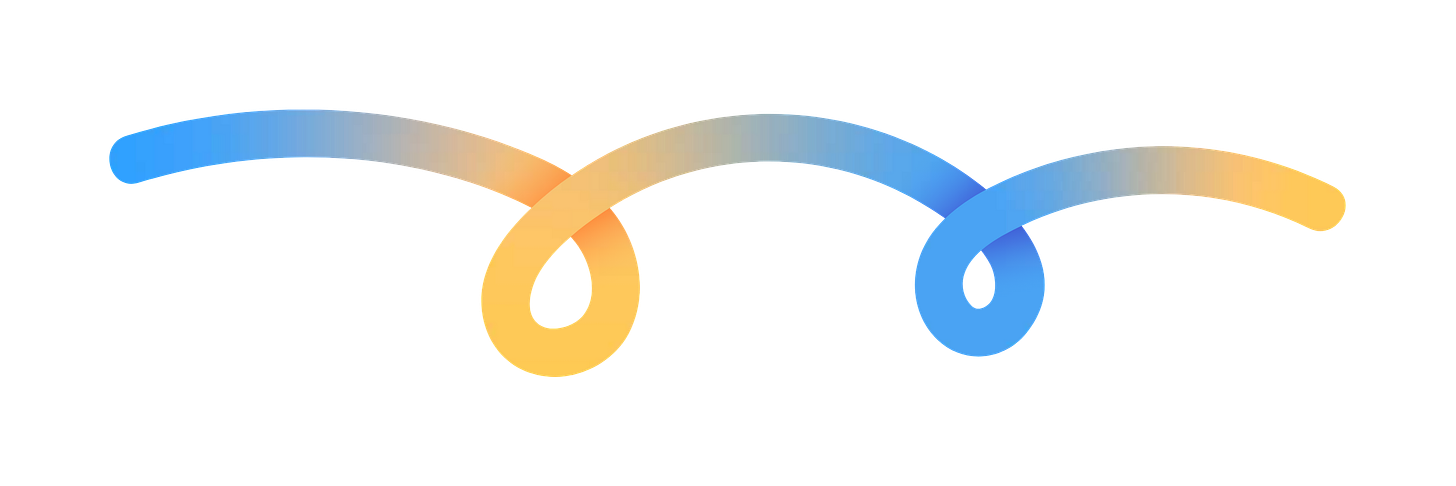
Generative AI:
While Generative AI is significant, it’s not the pinnacle of AI advancements. Beyond 2023, other AI innovations will share the spotlight.
Timing and Disruption:
Many AI innovations have existed for some time, but the current climate is ripe for their disruptive potential. The present is their moment to shine, and it’s crucial to leverage their capabilities now.
Leadership in Innovation:
Over time, being at the forefront of transformative innovations is essential, and the market recognizes and rewards such leadership. It’s pivotal to spot, invest in, and lead in these disruptive technologies early on to gain a competitive edge.